Making Room for Inclusion in AI-Generated Art: Combating Biases within Algorithms
By now, most of us have discovered the wonderful breakthroughs in AI-generated art. From a simple text prompt, AI engines such as Midjourney, Stable Diffusion, and DALL-E can generate entire scenes, and now short videos. Soon, we’ll have longer videos and likely entire procedurally-generated 3D metaverses. Unfortunately, the biases that have been inherent in AI for some time now, are– well– more visible than ever.
AI image generation works in a similar pattern to most common AI: a team will assemble a large amount of data sets (statistics, text, speech, images, video) and then spend time training the AI. This usually happens by having a team identify the target objects in each image (e.g. the cat sitting on a sofa, a cat sitting on a table) or statistically analyzing which words usually seem to be positioned next to each other (e.g. “peanut butter” is 90% likely to be followed with “jelly” but only 15% likely to be followed by “honey”). For generating art, these AI engines have been fed thousands of paintings, drawings, and photographs. These images were tagged, sometimes manually, but increasingly automatically, as the AI recognized objects (the AI knows what a cat looks like). These recognitions act cumulatively, so now an AI “knows” what a cat looks like, “knows” what a whiteboard looks like, and “knows” the visual patterns that are unique to Matisse, Dali, or Banksy.
These patterns, and the data sets from which they are derived, are the problem. Because of these three truths: (1) AI has been trained manually by human developers, (2) humans are inherently unconsciously biased, and (3) white men have been the majority identity of developers, it makes sense that AI-generated media favors whiteness and men. Let’s take a look at some examples of how AI favors these identities with simple, generic AI generated prompts, using Midjourney.
- When we type in /imagine a group of developers with laptops sitting around a conference table, Canon 200mm lens, wide focus –testp –upbeta we get the image on the bottom left. Notice that we only mentioned the word “developers” and the AI automatically rendered images of men, because “developers” = “men” according to how the AI was trained. In order to get images of women as developers, we’d have to clearly specify “women” in our text. The same is true for when we type in /imagine developers with computers wearing lab coats working on a big project, Sigma 50mm f22 –testp as seen on the bottom left images. Basically, the AI plays to stereotypes. The problem arises: unless we are very specific, the AI produces the same male-centered images, which will only reinforce gender stereotypes.
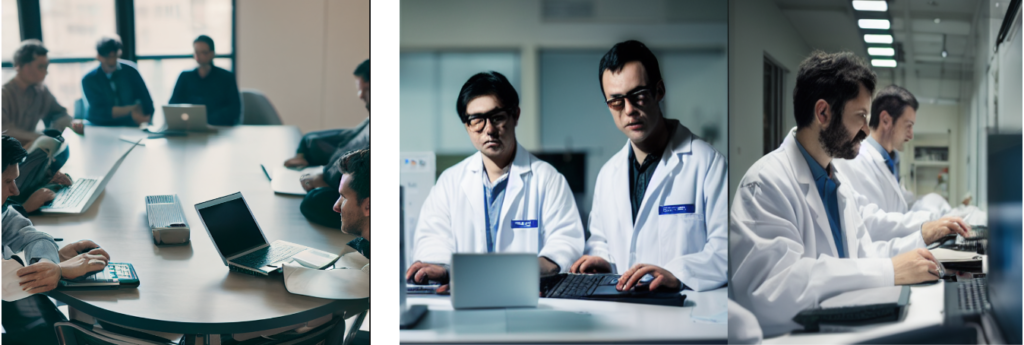
- To get past this, specificity is required: the prompt will do what it’s told, so it is up to the person entering the prompt to break the stereotypes. This is not ideal because it depends on a human to account for all possible biases in order to render an inclusive image. When we typed in /imagine 3 women developers with laptop computers on a card table inside an open garage, 50mm lens, f22 –testp we got the image on the bottom left. Similarly, when we typed in /imagine women data scientists working in a computer lab, in the style of a retro propaganda poster –ar 9:5 the images on the bottom right were developed. Notice that when typing “women” the AI automatically assumed “women” = “white.” This is another form of bias that intersects gender with race. Meaning, if we wanted images of women of Color (e.g. Black women, Indigenous women) we would need to be incredibly intricate with our descriptive words.
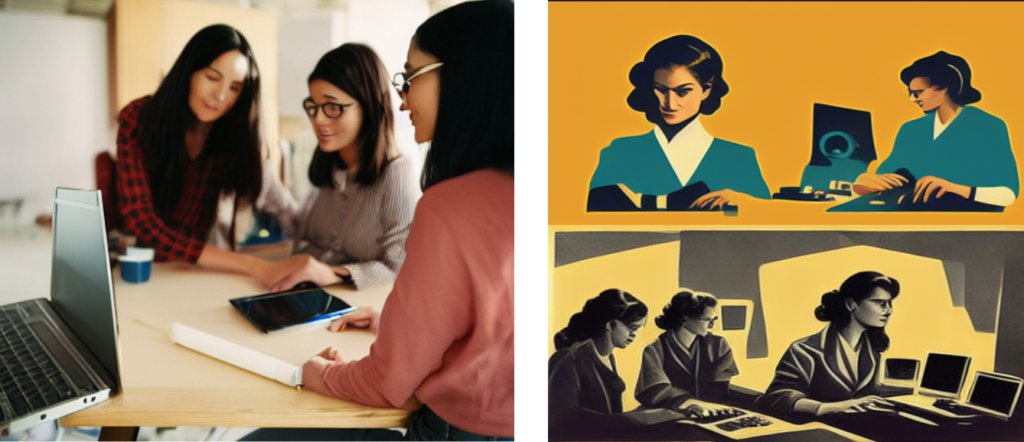
- Below, you’ll see AI-generated images that portrayed women of Color, once we were highly specific in our text prompts. On the bottom left, we typed in /imagine black women data scientists working in a computer lab, Canon 55mm lens, cinematic –ar 9:5, and on the bottom right, we typed in /imagine portrait of a Mexican woman with long curly hair, brown eyes, strong cheekbones, thick eyebrows, blush cheeks, Canon eos 5d mark III 85mm lens, headshot, photorealistic, hdr, 8k, cinematic lighting, closeup –testp.
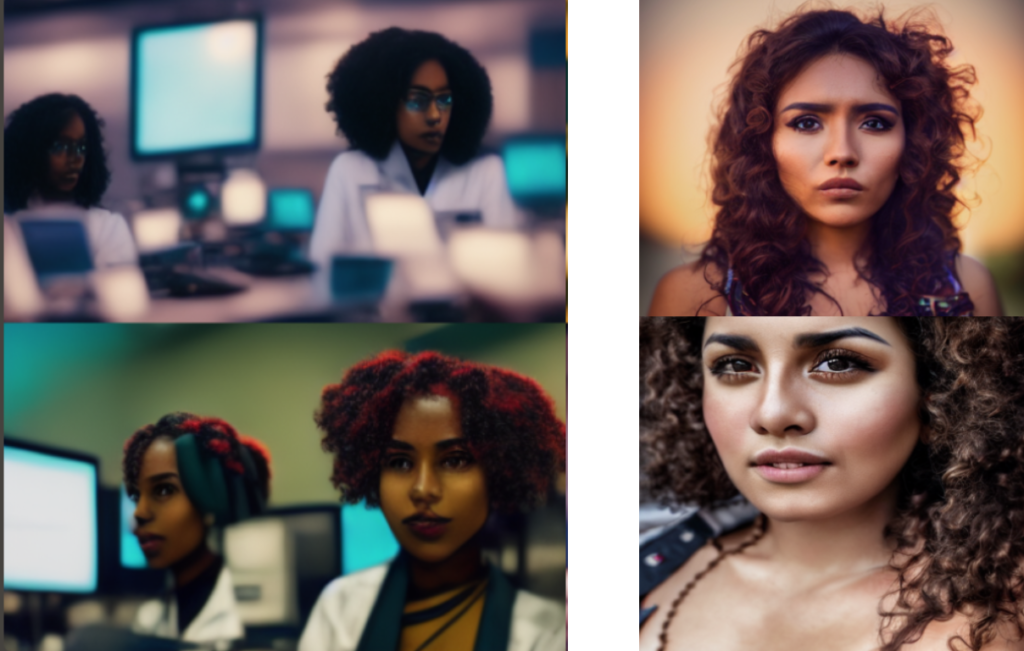
Just as in the real world, these biases reinforce societal norms that white men are the general standard and white women are the standard for women. Digitalization should be advancing us in all regards, including in diversity, equity, and inclusion efforts, not perpetuating harmful and outdated stereotypes. The good news is that biases can be overcome with forethought and intentional actions. At a programmatic level, it is key for teams building AI engines to identify and understand how biases creep into the AI, both from a cultural stereotyping perspective as well as the mathematical set of statistics upon which the AI draws its output.
It’s early days, still. The AI will improve in accuracy and creative ability. As AI continues to march into more areas of our lives, we will see an increasingly important role for “AI ethicist”, “AI bias manager”, and “AI culturalist”. With some effort, AI bias can be counteracted.